Figure 1: Most urban trips are shorter than 2.5 miles compared to suburban trips (under 4 miles).
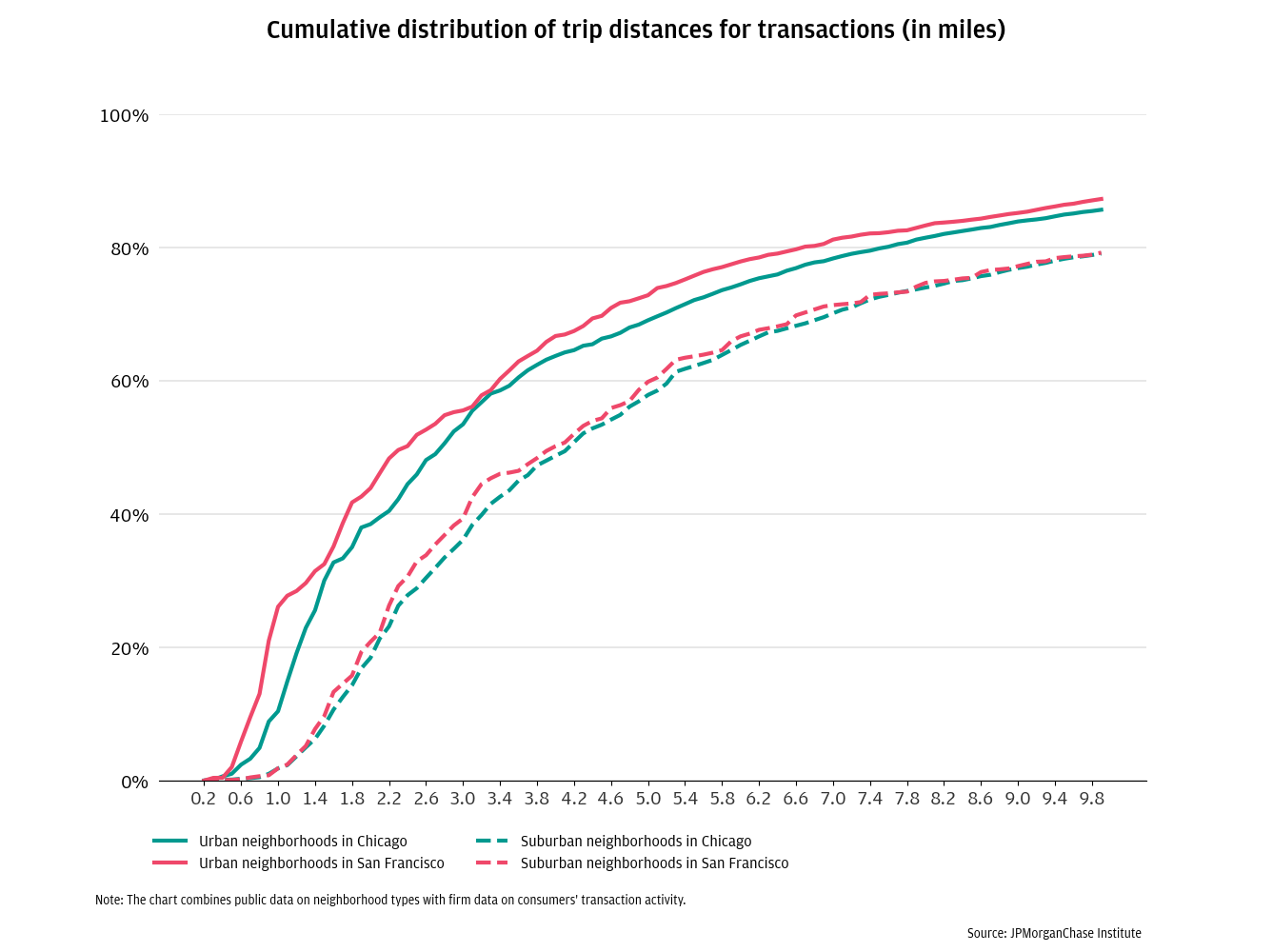
Research
December 18, 2024
Cities support economic growth by bringing people closer together, making it easier to access essential goods and services, and lowering costs for businesses to attract customers. However, some residents and neighborhoods benefit from this proximity more than others. Specifically, persistent segregation of neighborhoods by race and income has the potential to shape the proximity of consumers and retail businesses and the distribution of these economic benefits.
While recent research using administrative data suggests race and income may indeed play a role in shaping the movement of city residents outside their homes, schools, and jobs, it does not directly measure how race and income shape shopping destination choices across broad consumption categories. This report uses deidentified bank data to explore the interaction of race, income, distance, and shopping activity in Chicago and San Francisco—two metro areas with the highest levels of residential segregation in the U.S.
Our findings underscore significant disparities in access to retail shopping and services as well as related economic opportunities, demonstrating a need for better understanding of these issues and for targeted policy interventions focused on housing and business development and access to transportation.
Findings
Cities in which interactions are constrained by race and income risk both local pockets of economic stagnation and limited overall economic growth. In broad terms, cities provide innovation-driven economic growth by connecting people with different backgrounds and experiences (Glaser, et al. 1992). However, race and income shape everyday economic experiences in ways that can potentially undermine this promise. Specifically, people persistently live in neighborhoods that are segregated by race and income (Hwang and McDaniel 2022).1 Students living in these neighborhoods increasingly go to same-race, segregated schools (Reardon and Owens 2014), and workers most often work in persistently segregated workplaces (Tomaskovic-Devey, et al. 2006). The relative stability of housing, school, and work arrangements suggests that day-to-day interactions in these spheres provide limited exposure of residents to people of different backgrounds.
In principle, residents of metropolitan areas have more flexibility in the places they shop. Comparatively public retail spaces could draw together people from otherwise segregated daily experiences (Anderson 2011, Anderson 2014). Specifically, it is unclear whether residents of neighborhoods segregated by race or income shop in nearby neighborhoods with different racial or income profiles. While recent research using administrative data suggests race and income may indeed play a role in shaping the movement of city residents outside of their homes, schools, and jobs,2 research using these data does not directly measure how race and income shape shopping destination choices across broad consumption categories.3
To fill this gap, we use deidentified bank transaction and demographic data from a sample of over 1.2 million Chase customers in Chicago and San Francisco to study how consumer race and income shape everyday shopping trips.4 As cities with some of the highest levels of racial housing segregation in the U.S., Chicago and San Francisco have neighborhoods that vary substantially in racial and income composition, providing a context in which it may be easier to detect the impact of race and income on shopping trip outcomes. We use these data to examine two facets of how race and income shape retail outcomes within cities. First, we explore how race shapes the distances that consumers travel to retail goods and services. Then, we explore how the race and income characteristics of neighborhoods shape the likelihood that consumers make shopping trips to the retail businesses operating within them. We conclude with implications for policymakers seeking to broaden the base of economic growth through investments in housing, business growth, and access to transportation.
The distance consumers travel to make everyday purchases are a meaningful component of the overall cost of acquiring goods and services, both in terms of the cash cost of transit and the noncash cost of time spent travelling. While consumers’ increasing use of e-commerce platforms may improve access to different goods and retailers, most everyday shopping is still done in person, and take-up of e-commerce is unevenly spread across the population (Wheat, Duguid, et al. 2021). While consumers might choose to travel farther for specific goods if they do not prefer options closer to home, traveling greater distances for a purchase usually implies an overall reduction in economic well-being for consumers (Abbiasov, et al. 2022). Accordingly, differences in trip distances can reflect inequalities in well-being from consumption.
All else equal, consumers choose shorter trips for most everyday purchases when they can (Relihan 2024, Miyauchi, Nakajima and Redding 2021, Davis, et al. 2019). This general pattern is reflected in our data. Figure 1 shows the cumulative distribution of trip distances5 in both Chicago and San Francisco, for both urban and suburban customers.6 Urban consumers in both cities travel less than suburban consumers to make these purchases. In both cities, about 30 percent of urban trips are one mile or less, and most trips are less than 2.5 miles. While suburban consumers travel farther, it is still rare for consumers across both areas to make very long shopping trips. About 30 percent of suburban trips are less than two miles, and most are less than 4 miles.
Figure 1: Most urban trips are shorter than 2.5 miles compared to suburban trips (under 4 miles).
All else equal, consumers likely prefer shorter shopping trips, suggesting disparities for those who travel longer distances. With these differences as context, we first explore whether these trip distances vary by race.7 Figure 2 shows typical trip distances for residents of the Chicago and San Francisco metro areas in the fourth quarter of 2022 by race in both urban and suburban areas.8 The figure confirms the overall difference in typical shopping trip distances between urban and suburban areas shown in Figure 1—within race, shopping trips are longer for suburban consumers than for urban consumers. However, the benefit of shorter shopping trips within urban areas was not equally distributed across race. In both cities, White urban consumers had shorter shopping trips. Most trips made by White urban residents were under 2.4 miles in both cities. In contrast, most trips by Black urban residents were over 3.6 miles—nearly the distance of a typical trip in a suburban area. The racial pattern of shopping trip distances within urban areas for Hispanic and Asian residents differed between Chicago and San Francisco. In Chicago, the typical trip distance for Asian and Hispanic residents was closer to that of White residents, while in San Francisco, the typical trip distance for Asian and Hispanic residents was closer to that of Black residents.
Figure 2: Black urban consumers travel farther to purchase everyday goods and services than White urban consumers.
Overall, racial differences in trip distances were smaller in the suburbs than in urban areas for both cities. Hispanic suburban residents had the shortest shopping trips by race—most trips by Hispanic suburban residents of Chicago and San Francisco were under 4 miles. Suburban Asian residents generally took the longest trips—across cities, most trips by Asian suburban residents were over 4.2 miles.
These results suggest meaningful differences in economic well-being by race for consumers, businesses, and communities. Our data reflect observed trips, rather than trips consumers might have taken to otherwise preferred retail destinations farther from their homes. If consumers value proximity, then race-based differences in distances to preferred retail choices were likely larger than those shown here. Those differences imply disparity in travel costs for consumers and fewer customers for the businesses they might otherwise visit. Moreover, they suggest that the purported benefits of communities that provide retail amenities within walkable or short drivable distances may not be equally available to all.
The second question we pursue is the extent to which the racial and income composition of neighborhoods of retail destinations shape shopping trip choices. We first use descriptive statistics to explore whether consumers are unconditionally more likely to make shopping trips to neighborhoods in which residents of their own race or income are prevalent. We then build a model of shopping trip choice that considers distance and retail density in addition to the race and income of a consumer and the racial and income composition of the destination neighborhood. Estimates from this model inform the extent to which the racial and income composition of destination neighborhoods are shaping shopping choices, while considering their preferences to shop closer to home in places where retail choices are abundant. Overall, we find the racial and income composition of destination neighborhoods strongly influenced consumer shopping behavior.
To assess the unconditional likelihood of shopping trips to neighborhoods of varying racial composition, we split our sample by individual-level race and then aggregated the destinations of all the consumers in that group into quartiles, based on the percentage of residents of the same race in the destination neighborhood. This process allowed us to analyze the extent to which—for instance—Hispanic consumers are more likely to shop in neighborhoods with larger or smaller shares of Hispanic residents. Figure 3 shows the results of this analysis for consumers in both the Chicago and San Francisco metro areas in 2022.9
Figure 3: Consumers tended to shop in neighborhoods with more residents of their own race.
In this analysis, we labeled neighborhoods in the top two quartiles as “racially concentrated,” and neighborhoods in the top quartile as “highly racially concentrated.”10 Figure 3 shows that Asian, Black, and Hispanic residents of both Chicago and San Francisco carried out the overwhelming majority of their everyday transactions in racially concentrated neighborhoods, and, in most cases, in highly-concentrated neighborhoods. For example, Hispanic Chicago residents made 74 percent of their everyday transactions in Hispanic-concentrated neighborhoods, and 51 percent in highly Hispanic-concentrated neighborhoods.11 White residents of both metro areas distributed their transactions more evenly across neighborhoods. White consumers made 51 percent of their transactions in White-concentrated neighborhoods in Chicago, and 40 percent of their transactions in White-concentrated neighborhoods in San Francisco.
We then assessed the unconditional likelihood of shopping trips to neighborhoods of different income levels. We grouped consumers by the quintile of their 2022 take-home income and neighborhoods by their median 2022 household income, and then computed the share of trips from each group of consumers for each income category of neighborhood. Figure 4 presents the results of this analysis. The results suggest a relationship between income and shopping destinations. In both metro areas, lower income consumers were more likely to transact in lower income neighborhoods than higher income consumers, and higher income consumers were more likely to transact in higher income neighborhoods than lower income consumers.
Figure 4: Consumers were more likely to spend in neighborhoods with similar income profiles to their own.
Overall, these results show how the spatial distribution of residents combined with preferences to shop close to home can replicate patterns of residential segregation in shopping trip outcomes. Most Asian, Black, and Hispanic consumers in our sample live in neighborhoods where many of their neighbors are of the same race. For example, in Chicago, 81 percent of Black residents lived in highly Black-concentrated neighborhoods, 79 percent of Asian residents lived in highly Asian-concentrated neighborhoods, and 69 percent of Hispanic residents lived in highly Hispanic-concentrated neighborhoods.12 In contrast, the share of White residents in a neighborhood is less predictive of where White residents live. Only 19 percent of White Chicago residents live in highly White-concentrated neighborhoods. Given the prevalence of relatively short shopping trips, the racial distribution of people into neighborhoods is consistent with the patterns of shopping trips shown in Figures 3 and 4.
We next assess whether shopping trips choices are shaped by neighborhood racial and income characteristics above and beyond what might be explained by existing patterns of residential segregation and preferences to shop at nearby locations with dense retail options. We use a linear probability model to predict the probability that a consumer will visit a particular neighborhood. The model considers the distance from the consumer’s home neighborhood to the destination, as well as the share of the city’s retail located in the destination neighborhood.13 It accounts for the consumer’s race and income, as well as the median income and share of non-White residents in the destination neighborhood. It also captures the interaction between individual consumer race and non-White resident share of the destination neighborhood, which allows us to investigate differential sensitivity to non-White destination residents by consumer race.14
To illustrate the estimates from this model, Figures 5 and 6 plot the estimated likelihood for a consumer to shop in a typical neighborhood15 during a given month, conditional on the race of the consumer and either the racial composition of the neighborhood or its median income. Each chart presents the likelihood of a visit to a hypothetical predominantly White neighborhood with 75 percent White residents, a predominantly non-White neighborhood with 75 percent non-White residents, a hypothetical high-income neighborhood with a median income at the 75th percentile of ZIP codes in the metro area, and a hypothetical low-income neighborhood with a median income at the 25th percentile of ZIP codes in the metro area.
Figure 5 shows that in Chicago, consumers of all race groups were more likely to visit predominantly White neighborhoods compared to predominantly non-White neighborhoods equally far away with the same amount of existing retail and similar income profiles. This is a striking finding—it suggests that race, particularly a preference for shopping in predominantly White neighborhoods, is a factor beyond proximity and retail availability that shapes consumer choice. Comparing two equally distant neighborhoods with the same retail density and median income, 9.6 percent of White consumers made trips to retailers in predominantly White neighborhoods, and 7.1 percent made trips to retailers in predominantly non-White neighborhoods—a difference of 2.5 percentage points. Put differently, if a retailer in a predominantly White neighborhood received visits from 96 White shoppers in a quarter, a comparable retailer in a predominantly non-White neighborhood received visits from 71 White shoppers. Differences for shoppers of other races were meaningfully smaller but still substantial. For instance, Black consumers in Chicago were 0.9 percentage points less likely to visit a predominantly White neighborhood than a predominantly non-White one.
Figure 5 also illustrates the relatively muted effect of neighborhood income in Chicago. Holding racial composition of the destination constant, consumers in all race groups were more likely to make a shopping trip to a low-income neighborhood, but income differences were notably smaller than racial composition differences. Hispanic consumers in Chicago had the greatest difference by income, being 0.9 percentage points more likely to shop in a low-income neighborhood as compared to a high-income neighborhood. This difference was comparable to the smallest difference between predominantly White and Non-White neighborhoods we observed across race groups in Chicago.
Figure 5: Chicago consumers of all races were more likely to visit neighborhoods with greater shares of White residents.
Figure 6 shows estimated shopping trip likelihoods to neighborhoods in San Francisco. In contrast to what we observed in Chicago, Asian, Black, and Hispanic consumers were all more likely to visit comparable predominantly non-White neighborhoods. Among consumers from these groups, this tendency was strongest among Hispanic consumers, who were 1.3 percentage points more likely to visit a predominantly non-White neighborhood relative to a comparable predominantly White neighborhood. In contrast, White consumers were meaningfully more likely to visit comparable predominantly White neighborhoods. With a difference of 1.9 percentage points, White consumers showed a differential likelihood to visit predominantly White neighborhoods nearly as large as White consumers in Chicago.
Figure 6: White consumers in San Francisco were much more likely to visit predominantly White neighborhoods, while Black consumers were much less likely to visit high income neighborhoods.
Figure 6 also presents differences in trip likelihoods by destination median income. Particularly for Black consumers in San Francisco, destination income played a substantially more significant role in shaping trip likelihoods than in Chicago. Specifically, Black consumers in San Francisco were 2.2 percentage points more likely to shop in a low-income neighborhood compared to a high-income neighborhood. In contrast, Asian consumers made shopping trips to low- and high-income neighborhoods at nearly equal rates, with a difference of only 0.1 percentage point.
Overall, these results show that the racial and income characteristics of neighborhoods play a role in shaping shopping behavior above and beyond what can be explained by the differences in the spatial availability of retail. Holding constant considerations about how far consumers travel to shop at an establishment and the density of available establishments in a neighborhood, the share of non-White residents and neighborhood income were important determinants of shopping choices. The pattern of these results differed between Chicago and San Francisco, suggesting that other city-level institutional factors may play a role in determining the relative importance of race and income in retail behavior.
We found that income and particularly race play significant roles in shaping the consumption experiences in two major U.S. cities. Existing and persistent patterns of racial and income segregation in housing combined with incentives to shop near home produce materially different outcomes for Black, Asian, and Hispanic consumers compared to White consumers.
Our results offer evidence that can inform policymaker efforts to deliver inclusive economic growth within cities through reducing income and racial segregation. It is of course possible for policies intended to fully eliminate differences across neighborhoods and equalize access to distant customers to have unintended consequences. Tight-knit communities with largely local interactions might in some cases strengthen within-community economic ties (Jacobs 1961, Moreno, et al. 2021), and neighborhoods with high concentrations of low-income residents may provide economic growth opportunities for their residents (Husock 2021). However, exposure to poverty can have negative impacts on both economic and non-economic outcomes (Kling, Liebman and Katz 2007, Chetty, Hendren and Katz 2016), and there is at best mixed evidence that shows benefits for either workers or businesses in racially segregated neighborhoods (Xie and Gough 2011, Aguilera 2009). This suggests at least some scope for improved economic outcomes through targeted reductions to income and racial segregation.
More specifically, our findings suggest that addressing inequalities in shopping trip distances and likelihoods could broaden economic growth for individuals, the businesses they support, and the communities where they operate. First, consumers who travel farther to purchase goods and services pay the time and money costs of reaching their shopping destinations. While some of these costs might be offset by lower housing prices, housing markets would have to be unusually efficient to overcome all differences in consumer well-being from differences in trip distance differences. Second, businesses in neighborhoods systematically less likely to attract shoppers may have difficulty generating the revenue it takes to survive and grow. Reducing disparities above and beyond those attributable to fundamental drivers such as retail density and distance in the kinds of neighborhoods that attract consumers could reduce differences in outcomes for the businesses that operate there. Finally, the additional foot traffic associated with thriving retail businesses can generate spillover effects in a community, including but not limited to generating more demand for neighboring businesses and generally increasing community vibrancy. As a result, improvements along these lines could increase both commercial and residential property values.
While this study was not designed to test the efficacy of specific policy or product interventions, we offer the following implications with these broad considerations in mind:
We use deidentified credit and debit card transactions of over 1 million customers from the Chicago metro area and 237,000 customers from the San Francisco metro area for this study. We limit our focus to these cities to allow for more nuanced discussions of how different city-specific phenomena might affect results. We chose Chicago and San Francisco because they are among the most segregated metro areas in the country.17 An analysis of “experienced isolation,” which captures individuals’ exposure to diverse others in the places they visit over the course of their days, demonstrated that residential segregation and experienced isolation are highly correlated (Athey, et al. 2021). Moreover, the long-standing customer base Chase has in both these metro areas provides a more stable and representative sample.
To ensure these customers are part of our stable customer base, they must have at least one transaction in every month during Q4 2019 through Q4 2022. They must also have lived in the same ZIP code for each month of one quarter.18 While the customer’s home ZIP must be in the specific metro area, we allow for people to move ZIP across quarters.19 The change in typical shopping distances that may arise from moving to a new part of the metro area is a behavioral change we want to capture, especially with the hollowing out of urban cores during the first two years of the COVID pandemic (Wheat, Duguid, et al. 2023).
To enrich our understanding of how individual race and income relate to people’s shopping patterns across each city, we use third-party data to capture individuals’ race and use the inflows into customers’ deposit accounts to create a measure of their income for a year.20 We then group customers into income quintiles based on their home metro region. To understand relevant features of the neighborhoods in our study cities, we use data from the American Community Survey and County Business Patterns.21
We limit our analysis to in-person transactions whose destination is within the Chicago and San Francisco metro areas. We further limit our transactions to a subset of goods and services that are well-captured by card spending and that we consider “everyday” retail spending. These are clothing, general goods, groceries, home goods and services, leisure, personal care services, pharmacies, professional consumer services, and restaurants.22 These restrictions help focus our analysis on consumers’ day-to-day or week-to-week experiences of their own city.
We use a linear probability model to estimate the likelihood that a consumer will visit a given neighborhood in a quarter. Specifically, we estimate the equation:
where:
Table A1 presents descriptive statistics for each of these variables across the two metro areas in our study. Table A2 presents coefficient estimates and regression summary statistics for the full model in each city for Q4 2022.
Table A1: Sample Description, Q4 2022
Note: Unit of analysis in this table is a person-ZIP code—an opportunity for a trip to either occur or not occur.
Table A2: Regression estimates for the full model, Q4 2022
Note: *p<.05 **p<0.01 ***p<0.001
Abbiasov, Timur, Cate Heine, Edward L. Glaeser, Carlo Ratti, Sadegh Sabouri, Arianna Salazar Miranda, and Paolo Santi. 2022. The 15-Minute City Quantified Using Mobility Data. National Bureau of Economic Research.
Aguilera, Michael Bernabé. 2009. "Ethnic enclaves and the earnings of self-employed Latinos." Small Business Economics 33: 413-425. doi:10.1007/s11187-009-9206-6.
Anderson, Elijah. 2011. The Cosmopolitan Canopy: Race and Civility in Everyday Life. New York, NY: W.W. Norton & Co.
Anderson, Elijah. 2014. "The White Space." Sociology of Race and Ethnicity 1 (1): 10-21. doi:0.1177/2332649214561306.
Athey, Susan, Billy Ferguson, Matthew Gentzkow, and Tobias Schmidt. 2021. "Estimating Experienced Racial Segregation in U.S. Cities Using Large-Scale GPS Data." Proceedings of the National Academies of Science.
Bureau of Labor Statistics. 2023. Labor force characteristics by race and ethnicity, 2021. Bureau of Labor Statistics.
Chetty, Raj, Nathaniel Hendren, and Lawrence F. Katz. 2016. "The Effects of Exposure to Better Neighborhoods on Children: New Evidence from the Moving to Opportunity Experiment." American Economic Review 106 (4): 855-902. doi:10.1257/aer.20150572.
Davis, Donald R., Jonathan I. Dingel, Joan Monras, and Eduardo Morales. 2019. "How Segregated is Urban Consumption?" Journal of Political Economy 127 (4): 1684-1738.
Farrell, Diana, Chris Wheat, Marvin Ward, and Lindsay Relihan. 2020. The Early Impact of COVID-19 on Local Commerce. Washington, DC: JPMorganChase Institute. https://www.jpmorganchase.com/institute/research/cities-local-communities/early-impact-covid-19-local-commerce.
Farrell, Diana, Christopher Wheat, and Carlos Grandet. 2019. "Place Matters: Small Business Financial Health in Urban Communnities." https://www.jpmorganchase.com/institute/all-topics/business-growth-and-entrepreneurship/place-matters-small-business-financial-health-in-urban-communities.
Farrell, Diana, Christopher Wheat, and Chi Mac. 2018. "Growth, Vitality, and Cash Flows: High-Frequency Evidence from 1 Million Small Businesses." https://www.jpmorganchase.com/institute/all-topics/business-growth-and-entrepreneurship/report-growth-vitality-cash-flows.
Glaser, Edward L., Heidi D. Kallal, José A. Scheinkman, and Andrei Shleifer. 1992. "Growth in Cities." Journal of Political Economy 100 (6): 1126-1152. doi:10.1086/261856.
Husock, Howard. 2021. The Poor Side of Town: And Why We Need It. New York: Encounter Books.
Hwang, Jackelyn, and Tyler W. McDaniel. 2022. "Racialized Reshuffling: Urban Change and the Persistence of Segregation in the Twenty-First Century." Annural Review of Sociology 48: 397-419. doi:10.1146/annurev-soc-030420-014126.
Jacobs, Jane. 1961. The Death and Life of Great American Cities. New York: Random House.
Kling, Jeffrey R., Jeffrey B. Liebman, and Lawrence F. Katz. 2007. "Experimental Analysis of Neighborhood Effects." Econometrica 75 (1): 83-119.
Landis, John D. 2022. "Minority travel disparities and residential segregation: Evidence from the 2017 national household travel survey." Transportation Research Part D: Transport and Environment 112. doi:10.1016/j.trd.2022.103455.
Miyauchi, Yuhei, Kentaro Nakajima, and Stephen J. Redding. 2021. "Consumption Access and Agglomeration: Evidence from Smartphone Data." NBER Working Paper. http://www.nber.org/papers/w28497.
Moore, Ryan T., and Andrew Reeves. 2020. "Defining Racial and Ethnic Context with Geolocation Data." Political Science Research and Methods 8: 780-794. doi:10.1017/psrm.2020.10.
Reardon, Sean F., and Ann Owens. 2014. "60 Years After Brown: Trends and Consequences of School Segregation." Annual Review of Sociology 40: 199-214.
Reardon, Sean F., Stephen A Matthews, David O'Sullivan, Barret A. Lee, Glenn Firebuagh, Char R. Farrell, and Kendra Bischoff. 2008. "The Geographic Scale of Metropolitan Racial Segregation." Demography 45 (3): 489-514. doi:10.1353/dem.0.0019.
Relihan, Lindsay. 2024. "Clicks and Bricks: The Complementarity of Online Retail and Urban Services." London School of Economics CEP Discussion Paper. doi:10.2139/ss.
Schuetz, Jenny, Jed Kolko, and Rachel Meltzer. 2012. "Are poor neighborhoods "retail deserts"?" Regional Science and Urban Economics 42 (1-2): 269-285. doi:10.1016/j.regsciurbeco.2011.09.005.
Steil, Justin, and Michael Lens. 2023. "Public Policies To Address Residential Segregation And Improve Health." Health Affairs Health Policy Brief, April 27. doi:10.1377/hpb20230321.466701.
Tomaskovic-Devey, Donald, Catherine Zimmer, Kevin Stainback, Corre Robinson, Tiffany Taylor, and Tricia McTague. 2006. "Documenting Desegregation: Segregation in American Workplaces by Race, Ethnicity, and Sex, 1966-2003." American Sociological Review 71 (4): 565-588. doi:10.1177/000312240607100403.
University of California, Berkeley. 2023. Most to Least Segregated Metro Regions. https://belonging.berkeley.edu/most-least-segregated-metro-regions-2020.
Wang, Qi, Nolan Edward Phillips, Mario L. Small, and Robert J. Sampson. 2018. "Urban mobility and neighborhood isolation in America's 50 largest cities." Proceedings of the National Academy of Sciences 115 (30): 7735-7740. doi:10.1073/pnas.1802537115.
Wheat, Chris, James Duguid, Lindsay Relihan, and Bryan Kim. 2023. Downtown Downturn: The Covid Shock to Brick-and-Mortar Retail. Washington, DC: JPMorganChase Institute.
Wheat, Chris, James Duguid, Lindsay Relihan, and Bryan Kim. 2021. "The COVID Shock to Online Retail: The persistence of new online shopping habits and implications for the future of cities." JPMorganChase Institute. https://www.jpmorganchase.com/institute/research/cities-local-communities/covid-shock-to-online-retail.
Xie, Yu, and Margaret Gough. 2011. "Ethnic Enclaves and the Earnings of Immigrants." Demography 48 (4): 1293-1315. doi:10.1007/s13524-011-0058-8.
We thank our research team, Samantha Anderson and Peichen Li, for their hard work and contribution to this research. Additionally, we thank Oscar Cruz and Annabel Jouard for their support. We are indebted to our internal partners and colleagues, who support delivery of our agenda in a myriad of ways and acknowledge their contributions to each and all releases.
We are also grateful for the invaluable constructive feedback we received from external experts and partners. We are deeply grateful for their generosity of time, insight, and support.
We would like to acknowledge Jamie Dimon, CEO of JPMorgan Chase & Co., for his vision and leadership in establishing the Institute and enabling the ongoing research agenda. We remain deeply grateful to Peter Scher, Vice Chairman, Tim Berry, Head of Corporate Responsibility, Heather Higginbottom, Head of Research & Policy, and others across the firm for the resources and support to pioneer a new approach to contribute to global economic analysis and insight.
This material is a product of JPMorganChase Institute and is provided to you solely for general information purposes. Unless otherwise specifically stated, any views or opinions expressed herein are solely those of the authors listed and may differ from the views and opinions expressed by J.P. Morgan Securities LLC (JPMS) Research Department or other departments or divisions of JPMorgan Chase & Co. or its affiliates. This material is not a product of the Research Department of JPMS. Information has been obtained from sources believed to be reliable, but JPMorgan Chase & Co. or its affiliates and/or subsidiaries (collectively J.P. Morgan) do not warrant its completeness or accuracy. Opinions and estimates constitute our judgment as of the date of this material and are subject to change without notice. No representation or warranty should be made with regard to any computations, graphs, tables, diagrams or commentary in this material, which is provided for illustration/reference purposes only. The data relied on for this report are based on past transactions and may not be indicative of future results. J.P. Morgan assumes no duty to update any information in this material in the event that such information changes. The opinion herein should not be construed as an individual recommendation for any particular client and is not intended as advice or recommendations of particular securities, financial instruments, or strategies for a particular client. This material does not constitute a solicitation or offer in any jurisdiction where such a solicitation is unlawful.
Wheat, Chris and James Duguid. 2024. “Race, income, and retail outcomes in San Francisco and Chicago.” JPMorganChase Institute. https://www.jpmorganchase.com/institute/all-topics/business-growth-and-entrepreneurship/race-income-and-retail-outcomes-in-san-francisco-and-chicago
Footnotes
Notably, Hwang and McDaniel (2022) show that racial segregation in housing is especially persistent in suburban areas and has increased along some dimensions for Hispanic and Asian residents. We define segregation as the state of local areas (e.g. neighborhoods) having racial and/or income compositions that differ from the racial and/or income composition of the broader area (e.g. metropolitan area).
Recent research based on administrative data provides a distinctive lens on these trips (Moore and Reeves 2020). Social media activity data suggest that—across all types of activity—residents of mostly Black and Hispanic neighborhoods are unlikely to visit higher income or White middle-class neighborhoods (Wang, et al. 2018). Data from online reviews suggest that race plays a role in shaping restaurant choices in New York City, though perhaps less so than it does shaping housing choices (Davis, et al. 2019). This directional finding is reinforced by a broader study leveraging cellphone GPS data that suggests experienced Black-White isolation is less pronounced than residential isolation in cities such as New York, which are relatively dense, wealthy, highly educated, and have higher levels of public transit infrastructure (Athey, et al. 2021).
See Meltzer and Schuetz (2012), Kwate, et al. (2012), and Small and McDermott (2006) as examples.
See Appendix for more details about the data used in this study.
We measure distance as the Haversine distance between the centroids of the home and destination ZIP. When the home and destination ZIPs are the same, we calculate the distance of the transaction as 1/3 of the radius of a circle with the area of the home ZIP. This is to account for the fact that when customers travel within their home neighborhood, they travel some distance. We allow this distance to vary with the size of the ZIP code, as the size of ZIP codes increases moving further away from the center of a city. While similar studies have used a more detailed approach to measuring distance (Davis, et al. 2019), our approach provides distance estimates for the large number of trips across multiple cities in our data.
We define urban consumers as those with a home address in a ZIP code within Chicago or San Francisco city limits and suburban consumers as those with home addresses in all other ZIP codes in the CBSA.
We did not observe as pronounced differences when examining trip distances across income groups.
We also measured trip distances in Q4 2019. Urban and suburban residents of both Chicago and San Francisco made shorter shopping trips in 2022 than they did in 2019, across racial groups. In the wake of the COVID pandemic, commuting and shopping patterns changed, with more local trips and more online purchases (D. Farrell, C. Wheat, et al. 2020). The overall reductions we observed are consistent with this pattern, if generally small in magnitude, and unevenly distributed by race. The only groups that saw declines greater than 0.2 miles were Asian and White residents of the suburbs of both cities, and Asian and White urban residents of Chicago.
We also analyzed these patterns in Q4 2019 and found no substantive differences. Unconditional trip likelihoods by neighborhood race and income did not meaningfully change from before the COVID pandemic to 2022.
These are relative measures of concentration, as White residents are a much larger share of the population in both the Chicago and San Francisco metro areas. In Chicago, the median shares of Asian, Black, Hispanic, and White residents in a neighborhood were 2.8 percent, 3.9 percent, 11.8 percent, and 65.2 percent. In San Francisco those shares were 16 percent, 2.8 percent, 13.9 percent, and 46.1 percent.
The share of Hispanic residents of neighborhoods in the top quartile ranged between 22% and 93%.
In San Francisco, 74, 59, 60, and 17 percent of Black, Asian, Hispanic, and White residents lived in highly racially concentrated neighborhoods, respectively.
We consider retail density in our model, as it may vary with race and income. Prior research has shown that neighborhoods with lower-income residents and higher shares of Black and Hispanic residents have lower retail density, defined as the number of retail employees in a neighborhood divided by its spatial size (Schuetz, Kolko and Meltzer 2012).
We report details of the model specification, descriptive statistics, and regression estimates in the Appendix.
In both figures, the typical neighborhood is modeled as being 2.5 miles away, with a median income of $108,000. Estimates for Figure 5 assume that the typical neighborhood has 0.25% of all establishments—in Figure 6, we assume that the typical neighborhood has 0.39% of all establishments.
See Steil and Lens (2023) for a recent review.
In a study leveraging Census data to measure segregation using the divergence index, Chicago ranked first and San Francisco ranked twenty-second among the largest 225 metro areas in 2020 (University of California, Berkeley 2023).
This restriction makes measurement of the distance people travel to their shopping destinations more accurate. We measure the distance of a transaction as the distance between the centroid of the consumer’s home ZIP and the centroid of the destination ZIP. Customers who move during a quarter may move to a place where they have to meaningfully change their travel patterns. Take a customer who moves from the city to the suburbs for example. Grouping their transactions in a quarter during which they moved may give a misleading impression of their typical behavior.
We use the 2012 Core-Based Statistical Area definition of each metro area. Notably, this excludes San Jose from the San Francisco metro area.
We calculate a proxy for income by aggregating the inflows into customers’ checking accounts over the course of a year. Customers who have fewer than ten months of inflows and ten months of outflows into their checking accounts are dropped from our sample to guard against underestimating incomes of new customers whose activity we observe for only part of a year.
Specifically, we use the American Community Survey 2022 5-year Median Household Income estimates.
See notes in Figures 6 and 7 of Wheat, Duguid, et al. (2023) for more details on specific types of retailers in each category.
Authors
Chris Wheat
President, JPMorganChase Institute
James Duguid
Former Research Lead, JPMorganChase Institute